Reinforcement Learning from Human Feedback • RLHF
RLHF is a technique that uses reinforcement learning to optimize an AI agent's policy using human feedback. This technique has been successfully applied to various areas of natural language processing and video game bots, allowing agents to learn from human preferences and generate more natural and verbose responses. Reinforcement learning from human feedback (RLHF), also known as reinforcement learning from human preferences, is a machine learning technique that uses human feedback to optimize an agent's policy with reinforcement learning. A reward model is trained from human feedback and used as the reward function for the optimization algorithm. This technique can be used to improve the robustness and exploration of RL agents, particularly when the reward function is sparse or noisy. Human feedback is collected by asking humans to rank instances of the agent's behavior, which is then used to score outputs. RLHF has been successfully applied to natural language processing tasks and video game bots, allowing agents to learn from human preferences and generate more natural and verbose responses.Reinforcement learning from human feedback (RLHF) has been applied to natural language processing tasks, such as conversational agents, text summarization, and understanding of language. It is difficult to use ordinary reinforcement learning in these tasks because rewards are often difficult to define or measure. RLHF enables language models to use human preferences to provide answers that align with complex values and generate more verbose responses. Additionally, RLHF has been used to develop video game bots that have outperformed humans in various environments.Articles about RLHF
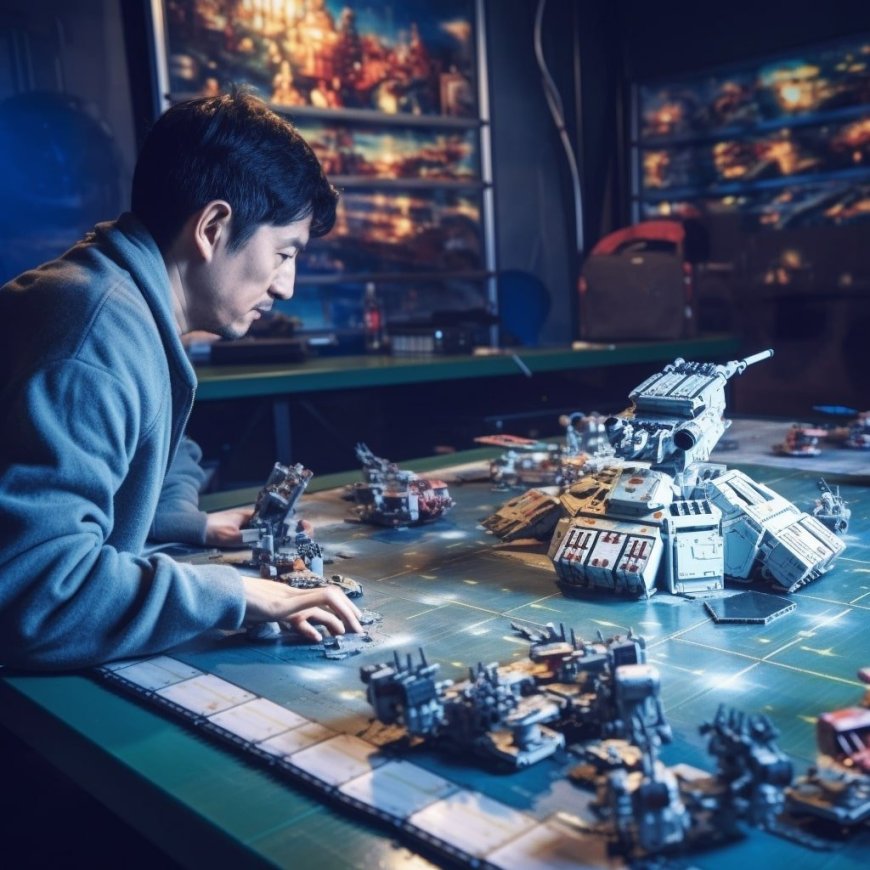
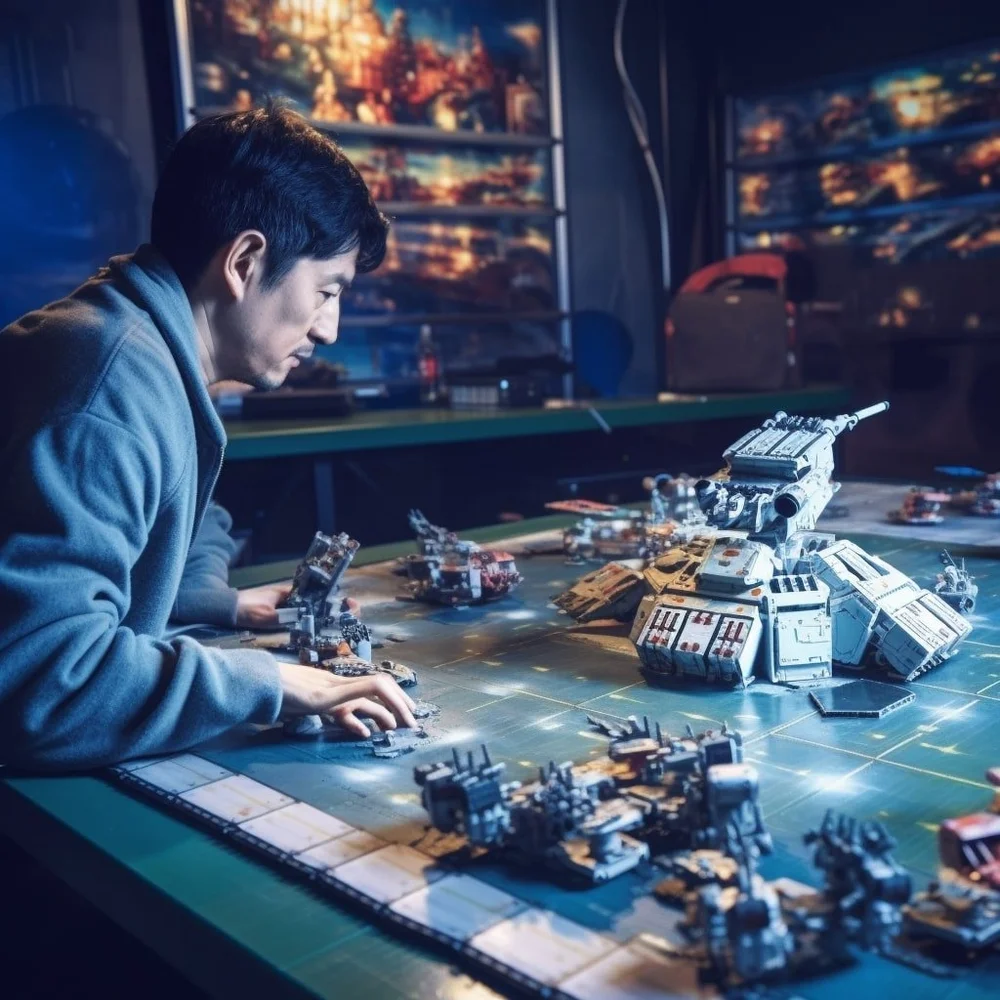
RLHF is a technique that uses reinforcement learning to optimize an AI agent's policy using human feedback. This technique has been successfully applied to various areas of natural language processing and video game bots, allowing agents to learn from human preferences and generate more natural and verbose responses.
Reinforcement learning from human feedback (RLHF), also known as reinforcement learning from human preferences, is a machine learning technique that uses human feedback to optimize an agent's policy with reinforcement learning. A reward model is trained from human feedback and used as the reward function for the optimization algorithm. This technique can be used to improve the robustness and exploration of RL agents, particularly when the reward function is sparse or noisy. Human feedback is collected by asking humans to rank instances of the agent's behavior, which is then used to score outputs. RLHF has been successfully applied to natural language processing tasks and video game bots, allowing agents to learn from human preferences and generate more natural and verbose responses.
Reinforcement learning from human feedback (RLHF) has been applied to natural language processing tasks, such as conversational agents, text summarization, and understanding of language. It is difficult to use ordinary reinforcement learning in these tasks because rewards are often difficult to define or measure. RLHF enables language models to use human preferences to provide answers that align with complex values and generate more verbose responses. Additionally, RLHF has been used to develop video game bots that have outperformed humans in various environments.